Measure theory
Table of Contents
Notation
and
are used to denote the indicator or characteristic function
Definition
Motivation
The motivation behind defining such a thing is related to the Banach-Tarski paradox, which says that it is possible to decompose the 3-dimensional solid unit ball into finitely many pieces and, using only rotations and translations, reassemble the pieces into two solid balls each with the same volume as the original. The pieces in the decomposition, constructed using the axiom of choice, are non-measurable sets.
Informally, the axiom of choice, says that given a collecions of bins, each containing at least one object, it's possible to make a selection of exactly one object from each bin.
Measure space
If is a set with the sigma-algebra
and the measure
, then we have a measure space .
Product measure
Given two measurable spaces and measures on them, one can obtain a product measurable space and a product measure on that space.
A product measure is defined to be a measure on the measurable space
, where we've let
be the algebra on the Cartesian product
. This sigma-algebra is called the tensor-product sigma-algebra on the product space, which is defined

A product measure is defined to be a measure on the measurable space
satisfying the property

and 
Let be a sequence of extended real numbers.
The limit inferior is defined

The limit supremum is defined

Premeasure
Given a space , and a collection of sets
is an algebra of sets on
if
- If
, then
- If
and
are in
, then
Thus, a algebra of sets allow only finite unions, unlike σ-algebras where we allow countable unions.
Given a space and an algebra
, a premeasure is a function
such that
For every finite or countable collection of disjoint sets
with
, if
then
Observe that the last property says that IF this "possibly large" union is in the algebra, THEN that sum exists.
A premeasure space is a triple where
is a space,
is an algebra, and a premeasure
.
Complete measure
A complete measure (or, more precisely, a complete measure space ) is a measure space in which every subset of every null set is measurable (having measure zero).
More formally, is complete if and only if

If is a premeasure space, then there is a complete measure space
such that
we have
If is σ-finite, then
is the only measure on
that is equal to
on
.
Atomic measure
Let be a measure space.
Then a set is called an atom if

and

A measure which has no atoms is called non-atomic or diffuse
In other words, a measure is non-atomic if for any measurable set
with
, there exists a measurable subset
s.t.

π-system
Let be any set. A family
of subsets of
is called a π-system if
If
, then
So this is an even weaker notion than being an (Boolean) algebra. We introduce it because it's sufficient to prove uniqueness of measures:
Theorems
Jensen's inequality
Let
be a probability space
be random variable
be a convex function Then
is the supremum of a sequence of affine functions
for
, with
.
Then is well-defined, and
![\begin{equation*}
\mathbb{E}[c(X)] \overset{a.s.}{\ge} a_n \mathbb{E}[X] + b_n
\end{equation*}](../../assets/latex/measure_theory_27722a1c52318e0443a664830effa0bb1a3503a3.png)
Taking the supremum over in this inequality, we obtain
![\begin{equation*}
\mathbb{E}[c(X)] \ge c \big( \mathbb{E}[X] \big)
\end{equation*}](../../assets/latex/measure_theory_8c3280bc7d5e2fce41ec6d17771166c73ae27475.png)
be a convex function
Then
is the supremum of a sequence of affine functions

Suppose is convex, then for each point
there exists an affine function
s.t.
- the line
corresponding to
passes through
- the graph of
lies entirely above
Let be the set of all such functions. We have
because
passes through the point
beacuse all
lies below
Hence
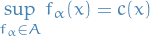
(note this is for each , i.e. pointwise).
Sobolev space
Notation
is an open subset of
denotes a infinitively differentiable function
with compact support
is a multi-index of order
, i.e.
Definition
Vector space of functions equipped with a norm that is a combination of norms of the function itself and its derivatoves to a given order.
Intuitively, a Sobolev space is a space of functions with sufficiently many derivatives for some application domain, e.g. PDEs, and equipped with a norm that measures both size and regularity of a function.
The Sobolev space spaces combine the concepts of weak differentiability and Lebesgue norms (i.e.
spaces).
For a proper definition for different cases of dimension of the space , have a look at Wikipedia.
Motivation
Integration by parst yields that for every where
, and for all infinitively differentiable functions with compact support
:

Observe that LHS only makes sense if we assume to be locally integrable. If there exists a locally integrable function
, such that

we call the weak
-th partial derivative of
. If this exists, then it is uniquely defined almost everywhere, and thus it is uniquely determined as an element of a Lebesgue space (i.e.
function space).
On the other hand, if , then the classical and the weak derivative coincide!
Thus, if , we may denote it by
.
Example
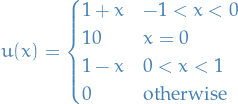
is not continuous at zero, and not differentiable at −1, 0, or 1. Yet the function
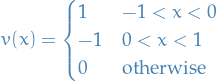
satisfies the definition of being the weak derivative of , which then qualifies as being in the Sobolev space
(for any allowed
).
Lebesgue measure
Notation
denotes the collection of all measurable sets
Stuff
Given a subset , with the length of a closed interval
given by
, the Lebesgue outer measure
is defined as

Lebesgue outer-measure has the following properties:
Idea: Cover by
.
(Monotinicy)
if
, then
Idea: a cover of
is a cover of
.
(Countable subadditivity) For every set
and every sequence of sets
if
then
Idea: construct a cover of each
,
such that
:
- Every point in
is in one of the
- Every point in
Q: Is it possible for every to find a cover
such that
?
A: No. Consider
. Given
, consider
.
This is a cover of
so
.
If
is a cover by open intervals of
, then there is at least one
such that
is a nonempty open interval, so it has a strictly positive lenght, and
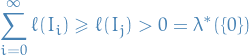
If , then
![\begin{equation*}
\lambda^* \big( [a, b] \big) = \lambda^*([a, b)) = \lambda^* \big( (a, b] \big) = \lambda^* \big( (a, b) \big)
\end{equation*}](../../assets/latex/measure_theory_e6c6a70aa01d16ddfce344bbaedabbd1746e51f5.png)
Idea: , so
.
For reverse, cover
by intervals giving a sum within
.
Then cover
and
by intervals of length
.
Put the 2 new sets at at the start of the sequence, to get a cover of
, and sum of the lengths is at most
. Hence,
![\begin{equation*}
\lambda^* \big( (a, b) \big) \le \lambda^* \big( [a, b] \big) \text{ and } \lambda^* \big( [a, b] \big) \le \lambda^* \big( (a, b) \big)
\end{equation*}](../../assets/latex/measure_theory_e07a5a74202cf48119137eaee61040e633e6ac87.png)
If is an open interval, then
.
Idea: lower bound from .
Only bounded nonempty intervals are interesting.
Take the closure to get a compact set. Given a countable cover by open intervals, reduce to a finite subcover.
Then arrange a finite collection of intervals in something like increasing order, possibly dropping unnecessary sets.
Call these new intervals
and let
be the number of such intervals, and such that

i.e. left-most interval cover the starting-point, and right-most interval cover the end-point. Then

Taking the infimum,

The Lebesgue measure is then defined on the Lebesgue sigma-algebra, which is the collection of all the sets which satisfy the condition that, for every

For any set in the Lebesgue sigma-algrebra, its Lebesgue measure is given by its Lebesgue outer measure .
IMPORTANT!!! This is not necessarily related to the Lebesgue integral! It CAN be be, but the integral is more general than JUST over some Lebesgue measure.
Intuition
- First part of definition states that the subset
is reduced to its outer measure by coverage by sets of closed intervals
- Each set of intervals
covers
in the sense that when the intervals are combined together by union, they contain
- Total length of any covering interval set can easily overestimate the measure of
, because
is a subset of the union of the intervals, and so the intervals include points which are not in
Lebesgue outer measure emerges as the greatest lower bound (infimum) of the lengths from among all possible such sets. Intuitively, it is the total length of those interval sets which fit most tightly and do not overlap.
In my own words: Lebesgue outer measure is smallest sum of the lengths of subintervals s.t. the union of these subintervals
completely "covers" (i.e. are equivalent to)
.
If you take an a real interval , then the Lebesge outer measure is simply
.
Properties
Notation
For
and
, we let
Stuff
The collection of Lebesgue measurable sets is a sigma-algebra.
Easy to see
is in this collection:
Closed under complements is clear: let
be Lebesgue measurable, then
hence this is also true for
, and so
is Lebesgue measurable.
- Closed under countable unions:
Finite case:
. Consider
both Lebesgue measurable and some set
. Since
is L. measurable:
Since
is L. measurable:
which allows us to rewrite the above equation for
:
Observe that
By subadditivity:
Hence,
Then this follows for all finite cases by induction.
Countable disjoint case: Let
, and
. Further, let
.
Hence
is L. measurable. Thus,
Since the
are disjoint
and
:
Let
and note that
. Thus, by indiction
Thus,
Taking
:
Thus,
is L. measurable if the
are disjoint and L. measurable!
- Countable (not-necessarily-disjoint) case:
If
are not disjoint, let
and let
, which gives a sequence of disjoint sets, hence the above proof applies.
Every open interval is Lebesgue measurable, and the Borel sigma-algebra is a subset of the sigma-algebra of Lebesgue measurable sets.
Want to prove measurability of intervals of the form .
Idea:
- split any set
into the left and right part
- split any cover in the same way
- extend covers by
to make them open
is a measure space, and for al intervals
, the measure is the length.
Cantor set
Define
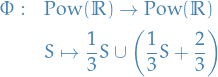
For , with
being identity, and

Let and
. Then the Cantor set is defined

The Cantor set has a Lebesgue measure zero.
We make the following observations:
- Scaled and shifted closed sets are closed
is a finite union of closed intervals and so is in the Borel sigma-algebra
- σ-algebras are closed under countable intersections, hence Cantor set is in the Borel σ-algebra
- Finally, Borel σ-algebra is a subset of Lebesgue measurable sets, hence the Cantor set is Lebesuge measurable!
Since Lebesgue measure satisfy for any Lebesgue measurable set
with finite measure and any
with
. Since Lebesgue measure is subadditive, we have for any

Since , by induction, it follows that
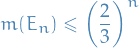
Taking the infimum of over , we have that the Cantor set has measure zero:

Cardinality of the Cantor set
Let .
The terniary expansion is a sequence with
such that
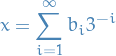
The Cantor set is uncountable.
We observe that if the first elements of the expansion for
are in
, then
. But importantly, observe that some numbers have more than one terniary expansion, i.e.

in the terniary expansion. One can show that a number if and only if
has a terniary expansion with no 1 digits. Hence, the Cantor set
is uncountable!
One can see that if and only if terniary expansion with no 1 digits, since such an
would land in the "gaps" created by the construction of the Cantor set.
Uncountable Lebesgue measurable set
There exists uncountable Lebesgue measurable sets.
Menger sponge
- Generalization of Cantor set to
Vitali sets
Let if and only if
.
- There are uncountable many equivalence classes, with each equivalence class being countable (as a set).
- By axiom of choice, we can pick one element from each equivalence class.
- Can assume each representative picked is in
, and this set we denote
Suppose, for the sake of contradiction, that is measurable.
Observe if , then there is a
and
s.t.
, i.e.
![\begin{equation*}
[0, 1] \subseteq \bigcup_{q \in [-1, 1] \cap \mathbb{Q}}^{} \Big( R + q \Big) \subseteq [-1, 2]
\end{equation*}](../../assets/latex/measure_theory_f79853c87366563f923b51372436ae537e0d78ed.png)
Then, by countable additivity
![\begin{equation*}
\begin{split}
m([0, 1]) &\le m \bigg( \bigcup_{q \in [-1, 1] \cap \mathbb{Q}}^{} R + q \bigg) \le m \big( [-1, 2] \big) = 3 \\
m([0, 1]) &\le \sum_{q \in [-1, 1] \cap \mathbb{Q}}^{} m (R + q) \le 3 \\
m([0, 1]) &\le \sum_{q \in [-1, 1] \cap \mathbb{Q}}^{} m (R) \le 3
\end{split}
\end{equation*}](../../assets/latex/measure_theory_10cc4bf4c4028ae61e6bec3e9a6df729d34e627e.png)
where we've used
![\begin{equation*}
m \bigg( \bigcup_{q \in [-1, 1] \cap \mathbb{Q}}^{} R + q \bigg) = \sum_{q \in [-1, 1] \cap \mathbb{Q}}^{} m (R + q) = \sum_{q \in [-1, 1] \cap \mathbb{Q}}^{} m (R)
\end{equation*}](../../assets/latex/measure_theory_4c878026d358487ad76ea895c4e45d05d969e74a.png)
Hence, we have our contradiction and so this set, the Vitali set, is not measurable!
There exists a subset of that is not measurable wrt. Lebesgue measure.
Lebesgue Integral
The Lebesgue integral of a function over a measure space
is written

which means we're taking the integral wrt. the measure .
Special case: non-negative real-valued function
Suppose that is a non-negative real-valued function.
Using the "partitioning of range of " philosophy, the integral of
should be the sum over
of the elementary area contained in the thin horizontal strip between
and
, which is just

Letting

The Lebesgue integral of is then defined by
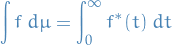
where the integral on the right is an ordinary improper Riemann integral. For the set of measurable functions, this defines the Lebesgue integral.
Radon measure
- Hard to find a good notion of measure on a topological space that is compatible with the topology in some sense
- One way is to define a measure on the Borel set of the topological space
Let be a measure on the sigma-algebra of Borel sets of a Hausdorff topological space
.
is called inner regular or tight if, for any Borel set
,
is the supremum of
over all compact subsets of
of
, i.e.
where
denotes the compact interior, i.e. union of all compact subsets
.
is called outer regular if, for any Borel set
,
is the infimum of
over all open sets
containing
, i.e.
where
denotes the closure of
.
is called locally finite if every point of
has a neighborhood
for which
is finite (if
is locally finite, then it follows that
is finite on compact sets)
The measure is called a Radon measure if it is inner regular and locally finite.
Suppose and
are two
measures on a measures on a measurable space
and
is absolutely continuous wrt.
.
Then there exists a non-negative, measurable function on
such that
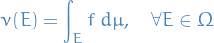
The function is called the density or Radon-Nikodym derivative of
wrt.
.
If a Radon-Nikodym derivative of wrt.
exists, then
denotes the equivalence class of measurable functions that are Radon-Nikodym derivatives of
wrt.
.
is often used to denote
, i.e.
is just in the equivalence class of measurable functions such that this is the case.
This comes from the fact that we have
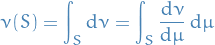
Suppose and
are Radon-Nikodym derivatives of
wrt.
iff
.
The δ measure cannot have a Radon-Nikodym derivative since integrating gives us zero for all measurable functions.
Continuity of measure
Suppose and
are two sigma-finite measures on a measure space
.
Then we say that is absolutely continuous wrt.
if

We say that and
are equivalent if each measure is absolutely continuous wrt. to the other.
Density
Suppose and
are two sigma-finite measures on a measure space
and that
is absolutely continuous wrt.
. Then there exists a non-negative, measurable function
on
such that
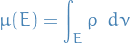
Measure-preserving transformation
is a measure-preserving transformation is a transformation on the measure-space
if

Measure
A measure on a set is a systematic way of defining a number to each subset of that set, intuitively interpreted as size.
In this sense, a measure is a generalization of the concepts of length, area, volume, etc.
Formally, let be a
of subsets of
.
Suppose is a function. Then
is a measure if
Whenever
are pairwise disjoint subsets of
in
, then
- Called σ-additivity or sub-additivity
Properties
Let be a measure space, and
such that
.
Then .
Let

Then , and by finite additivity property of a measure:

since by definition of a measure.
If are
subsets of
, then
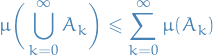
We know for a sequence of disjoint sets we have
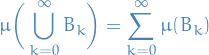
So we just let
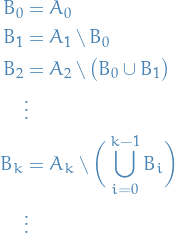
Then,
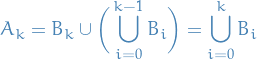
Thus,
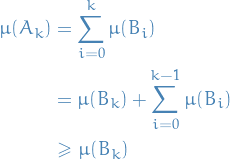
Concluding our proof!
Let be an increasing sequence of measurable sets.
Then
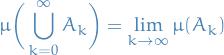
Let be sets from some
.
If , then
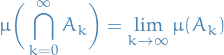
Examples of measures
Let
be a space
The δ-measure (at ) is
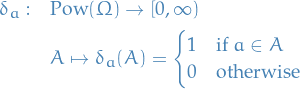
Sigma-algebra
Definition
Let be some set, and let
be its power set. Then the subset
is a called a σ-algebra on
if it satisfies the following three properties:
is closed under complement: if
is closed under countable unions: if
These properties also imply the following:
is closed under countable intersections: if
Generated σ-algebras
Given a space and a collection of subsets
, the σ-algebra generated by
, denoted
, is defined to be the intersection of all σ-algebras on
that contain
, i.e.
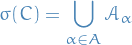
where

Let be a measurable space and
a function from some space
to
.
The σ-algebra generated by is

Observe that though this is similar to σ-algebra generated by MEASURABLE function, the definition differs in a sense that the preimage does not have to be measurable. In particular, the σ-algebra generated by a measurable function can be defined as above, where is measurable by definition of
being a measurable function, hence corresponding exactly to the other definition.
Let and
be measure spaces and
a measurable function.
The σ-algebra generated by is

Let be a space.
If is a collection of σ-algebras, then
is also a σ-algebra.
σ-finite
A measure or premeasure space is finite if
.
A measure on a measure space
is said to be sigma-finite if
can be written as a countable union of measurable sets of finite measure.
Example: counting measure on uncountable set is not σ-finite
Let be a space.
The counting measure is defined to be such that

On any uncountable set, the counting measure is not σ-finite, since if a set has finite counting measure it has countably many elements, and a countable union of finite sets is countable.
Properties
Let be a
of subsets of a set
. Then
If
, then
- If
then
Borel sigma-algebra
Any set in a topological space that can be formed from the open sets through the operations of:
- countable union
- countable intersection
- complement
is called a Borel set.
Thus, for some topological space , the collection of all Borel sets on
forms a σ-algebra, called the Borel algebra or Borel σ-algebra .
More compactly, the Borel σ-algebra on is

where is the σ-algebra generated by the standard topology on
.
Borel sets are important in measure theory, since any measure defined on the open sets of a space, or on the closed sets of a space, must also be defined on all Borel sets of that space.
Any measure defined on the Borel sets is called a Borel measure.
Lebesgue sigma-algebra
Basically the same as the Borel sigma-algebra but the Lebesgue sigma-algebra forms a complete measure.
Note to self
Suppose we have a Lebesgue mesaure on the real line, with measure space .
Suppose that is non-measurable subset of the real line, such as the Vitali set. Then the
measure of
is not defined, but

and this larger set ( ) does have
measure zero, i.e. it's not complete !
Motivation
Suppose we have constructed Lebesgue measure on the real line: denote this measure space by . We now wish to construct some two-dimensional Lebesgue measure
on the plane
as a product measure.
Naïvely, we could take the sigma-algebra on to be
, the smallest sigma-algebra containing all measureable "rectangles"
for
.
While this approach does define a measure space, it has a flaw: since every singleton set has one-dimensional Lebesgue measure zero,

for any subset of .
What follows is the important part!
However, suppose that is non-measureable subset of the real line, such as the Vitali set. Then the
measure of
is not defined (since we just supposed that
is non-measurable), but

and this larger set ( ) does have
measure zero, i.e. it's not complete !
Construction
Given a (possible incomplete) measure space , there is an extension
of this measure space that is complete .
The smallest such extension (i.e. the smallest sigma-algebra ) is called the completion of the measure space.
It can be constructed as follows:
- Let
be the set of all
measure zero subsets of
(intuitively, those elements of
that are not already in
are the ones preventing completeness from holding true)
- Let
be the sigma-algebra generated by
and
(i.e. the smallest sigma-algreba that contains every element of
and of
)
has an extension to
(which is unique if
is sigma-finite), called the outer measure of
, given by the infimum

Then is a complete measure space, and is the completion of
.
What we're saying here is:
- For the "multi-dimensional" case we need to take into account the zero-elements in the resulting sigma-algebra due the product between the 1D zero-element and some element NOT in our original sigma-algebra
- The above point means that we do NOT necessarily get completeness, despite the sigma-algebras defined on the sets individually prior to taking the Cartesian product being complete
- To "fix" this, we construct a outer measure
on the sigma-algebra where we have included all those zero-elements which are "missed" by the naïve approach,
Measurable functions
Let and
be measurable spaces.
A function is a measurable function if

where denotes the preimage of the
for the measurable set
.
Let .
We define the indicator function of to be the function
given by
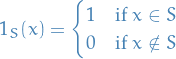
Let . Then
is measurable if and only if
.
Let be a measure space or a probability space.
Let be a sequence of measurable functions.
- For each
, the function
is measurable
- The function
is measurable
- Thus, if
converge pointwise,
is measurable.
Let be a measurable space, and let
.
The following statements are equivalent:
is measurable.
we have
.
we have
.
we have
.
we have
.
A function is measurable if
![\begin{equation*}
\forall c \in [- \infty, \infty) : f^{-1} \Big( (c, \infty] \Big) \in \mathcal{A}
\end{equation*}](../../assets/latex/measure_theory_cf13df725bd4ef04ae4396b0f5bcc9e8fdddb4bf.png)
We also observe that by Proposition proposition:equivalent-statements-to-being-a-measurable-function, it's sufficient to prove
![\begin{equation*}
\forall c \in (-\infty, \infty) : f^{-1} \big( [c, \infty] \big) \in \mathcal{A}
\end{equation*}](../../assets/latex/measure_theory_8e5e1bcca38b5b97bece913a91079e5c8dbc7a7d.png)
so that's what we set out to do.
For and
, consider the following equivalent statements:
![\begin{equation*}
\begin{align*}
& & x &\in \bigg( \inf_{n \ge m} f_n \bigg)^{- 1} \Big( [c, \infty] \Big) \\
& & \inf_{n \ge m} f_n(x) &\in [c, \infty] \\
& & \inf_{n \ge m} f_n(x) &\ge c \\
&\forall n \ge m : & f_n(x) &\ge c \\
& \forall n \ge m : & x & \in f_n^{-1} \big( [c, \infty \big) \\
& & x & \in \bigcap_{n \ge m}^{\infty} f_n^{-1} \big( [c, \infty] \big)
\end{align*}
\end{equation*}](../../assets/latex/measure_theory_94af009dc1af4db3187dfbf9a593271d7e6f84f7.png)
Thus,
![\begin{equation*}
\Big( \inf_{n \ge m} f_n \Big)^{-1} \big( [c, \infty] \big) = \bigcap_{n \ge m}^{} f_n^{-1} \big( [c, \infty] \big)
\end{equation*}](../../assets/latex/measure_theory_50a948d91ff66fee7b9175dd150be89b0439004f.png)
so
![\begin{equation*}
\big( \inf_{n \ge m} f_n \big)^{-1} \big( [c, \infty] \big) \in \mathcal{A}
\end{equation*}](../../assets/latex/measure_theory_e82c9dc08e0706905a550f6f6dcfba1d845ac5bb.png)
Recall that for each , the sequence
is an increasing sequence in
. Therefore, similarily, the following are equivalent:
![\begin{equation*}
\begin{align}
& & x & \in \big( \liminf_{n \to \infty} f_n \big)^{- 1} \big( [c, \infty] \big) \\
& & \liminf_{n \to \infty} f_n(x) & \in [c, \infty] \\
& & \uparrow \lim_{m \to \infty} \inf_{n \ge m} f_n(x) &\ge c \\
& & \sup_m \inf_{n \ge m} f_n(x) &\ge c \\
& \forall N \in \mathbb{Z} : \exist m \in \mathbb{N} & \inf_{n \ge m} f_n(x) &\ge c - \frac{1}{N} \\
& \forall N \in \mathbb{N} : & x &\in \bigcup_{m \in \mathbb{N}}^{} \bigg( \inf_{n \ge m} f_n \bigg)^{-1} \bigg( \bigg[ c - \frac{1}{N}, \infty \bigg] \bigg) \\
& & x & \in \bigcap_{N \in \mathbb{N}}^{} \bigcup_{m \in \mathbb{N}}^{} \bigg( \inf_{n \ge m} f_n \bigg)^{-1} \bigg( \bigg[ c - \frac{1}{N}, \infty \bigg] \bigg)
\end{align}
\end{equation*}](../../assets/latex/measure_theory_caecf37c6ca6765e01f08e6648c9e28ae668ffe0.png)
Thus,
![\begin{equation*}
\big( \liminf f_n \big)^{-1} \big( [c, \infty] \big) = \bigcap_{N \in \mathbb{N}}^{} \bigcup_{m \in \mathbb{N}}^{} \bigg( \inf_{n \ge m} f_n \bigg)^{-1} \bigg( \bigg[ c - \frac{1}{N}, \infty \bigg] \bigg)
\end{equation*}](../../assets/latex/measure_theory_c759a8999f8697bf52bd6d4e35f493d7ca24ec11.png)
Hence,
![\begin{equation*}
\big( \liminf f_n \big)^{-1} \big( [c, \infty] \big) \in \mathcal{A}
\end{equation*}](../../assets/latex/measure_theory_e6028aa99aabfafc829083191d1bf45609989c03.png)
concluding our proof!
Basically says the same as Prop. proposition:limits-of-measurable-functions-are-measurable, but a bit more "concrete".
Let be a
of subsets of a set
, and let
with
be a sequence of measurable functions.
Furthermore, let

Then is a measurable function.
Simple functions
Let be a
of subsets of a set
.
A function is called a simple function if
- it is measurable
- only takes a finite number of values
Let be a
of subsets of a set
.
Let be a nonnegative measurable function.
Then there exists a sequence of simple functions such that
for all
Converges to
:
Define a function as follows. Let

and let

Then the function
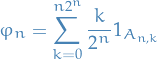
obeys the required properties!
Almost everywhere and almost surely
Let be a measure or probability space.
Let be a sequence of measurable functions
- For each
the function
is measurable
- The function
is measurable
- Thus, if the
converge pointwise, then
is measurable
Let be a measure space. Let
be a condition in oe variable.
holds almost everywhere (a.e.) if

Let be a probability space and
be a condition in one variable, then
holds almost surely (a.e.) if

also denoted

Let be a complete measure space.
- If
is measurable and if
a.e. then
is measurable.
- Being equal a.e. is an equivalence relation on measurable functions.
Convergence theorems for nonnegative functions
Problems
Clearly if with
s.t.
, then

hence

Therefore it's sufficient to prove that if , then there exists a non-degenerate open interval
s.t.
. (first I said contained in
, but that is a unecessarily strong statement; if contained then what we want would hold, but what we want does not imply containment).
As we know, for every there exists
such that
and

Which implies

which implies

Letting , this implies that there exists an open cover
s.t.

and

(this fact that this is true can be seen by considering for all
and see that this would imply
not being a cover of
, and if
, then since
there exists a "smaller" cover).
Thus,

Hence, letting be s.t.

we have

as wanted!
, we have
for almost every
if and only if for almost every
,
for all
.
This is equivalent to saying
![\begin{equation*}
m \Big( f_n^{-1} \big( (-\infty, 0] \big) \Big) = 0, \quad \forall n \in \mathbb{N}
\end{equation*}](../../assets/latex/measure_theory_67584d2b69d979eee0f59c423a7e6cfbc7c4b82a.png)
if and only if
![\begin{equation*}
m \bigg( \bigcup_{n = 0}^{\infty} f_n^{-1} \Big( (-\infty, 0] \Big) \bigg) = 0
\end{equation*}](../../assets/latex/measure_theory_4acf342f12fd15b4c6d3b130fe41fb4825810116.png)
i.e. is a set of measure zero.
Then clearly
![\begin{equation*}
m \bigg( \bigcup_{n=0}^{\infty} f_n^{-1} \big( (- \infty, 0] \big) \bigg) = \sum_{n=0}^{\infty} \underbrace{m \Big( f_n^{-1} \big( (- \infty, 0] \big) \Big)}_{= 0} = 0
\end{equation*}](../../assets/latex/measure_theory_b5c3b4a7db26f3d66f1400bc53d458741769e23b.png)
by the assumption.
Follows by the same logic:
![\begin{equation*}
\sum_{n=0}^{\infty} m \Big( f_n^{-1} \big( (- \infty, 0] \big) \Big) = \underbrace{m \bigg( \bigcup_{n=0}^{\infty} f_n^{-1} \big( (- \infty, 0] \big) \bigg)}_{= 0} = 0
\end{equation*}](../../assets/latex/measure_theory_6b06dbd7651e8132421b8a66be9d25590a352936.png)
This concludes our proof.
Integration
Notation
We let
where
Stuff
Let
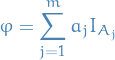
where are a set of positive values.
Then the integral of
over
wrt.
is given by
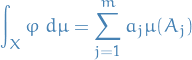
Let be a sequence of nonnegative measurable functions on
. Assume that
for each
for each
.
Then, we write pointwise.
Then is measurable, and

Let . By Proposition proposition:limit-of-measurable-functions-is-measurable,
is measurable.
Since each satisfies
, we know
.
- If
, then since
and for all
we have
, and
.
Let and
.
Step 1: Approximate by a simple function.
Let be a simple function such that
and
.
Such an
exists by definition of Lebesgue integral. Thus, there are
such that
, and disjoint mesurable sets
such that
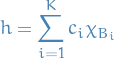
If any , it doesn't contribute to the integral, so we may ignore it and assume that there are no such sets.
Step 2: Find sets of large measure where the convergence is controlled.
Note that for all we have

That is, for each and
,

For and
, let

And since it's easier to work with disjoint sets,
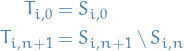
Observe that,
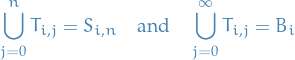
Then,
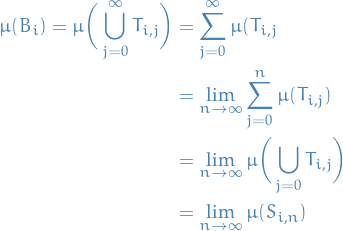
We don't have a "rate of convergence" on , but on
we know that we are
close, and so we can "control" the convergence.
Step 3: Approximate from below.
For each if
, then let
be such that

and otherwise, let be such that
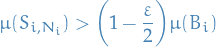
Let , and let
.
For each ,
and
we have

Thus, , and
,
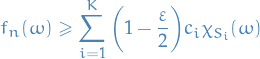
If there is a such that
, then
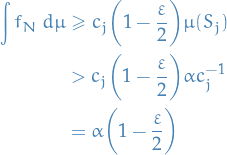
Otherwise (if the integral is finite), then
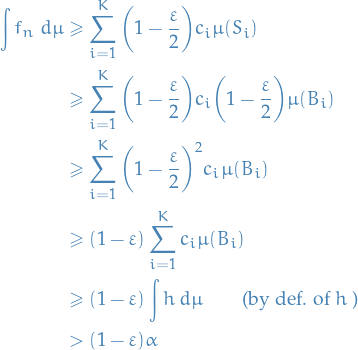
For every and
, there is an
such that

For every such that
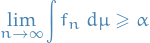
Therefore
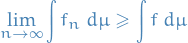
Thus,

as wanted.
Let be any nonnegative measurable functions on
.
Then

Let and observe
are pointwise increasing
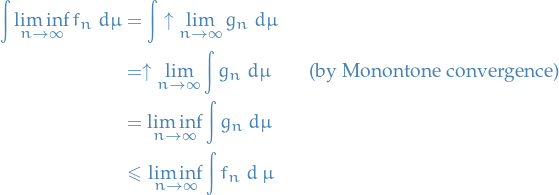
Properties of integrals
Let be a measure space.
If is a nonnegative measurable function, then there is an increasing sequence of simple functions
such that
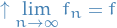
Given as above and
for
, let
![\begin{equation*}
\begin{split}
S_{n, k} &= f^{-1} \Big( \big[k 2^{-n}, (k + 1) 2^{-n} \big) \Big) \\
S_{n, 4^n} &= f^{-1} \Big( [2^n, \infty] \Big)
\end{split}
\end{equation*}](../../assets/latex/measure_theory_aa6b395505b8bd8701e0668288bf3f46245d7906.png)
and
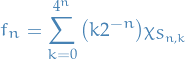
Or a bit more explicit (and maybe a bit clearer),
![\begin{equation*}
f_n = \underbrace{4^n 2^{-n}}_{= 2^n} \chi_{f^{-1} \Big( [2^n, \infty] \Big)} + \sum_{k=0}^{4^n - 1} \big( k 2^{-n} \big) \chi_{f^{-1} \Big( \big[\frac{k}{2^n}, \frac{k + 1}{2^n} \big) \Big)
\end{equation*}](../../assets/latex/measure_theory_bc744558768b4a547f739ded454eaedaafbc4971.png)
For each ,
is a cover of
. On each
we have
, hence
on entirety of
.
Consider . If
, then for
which in turn implies

Hence .
Finally, if , then
and for all
take on values

Hence, for all cases.
Furthermore, for any and
, there is the nesting property

so on we have
.
(This can be seen by observing that what we're really doing here is dividing the values takes on into a grid, and observing that if we're in
then we're either in
or
).
For , then
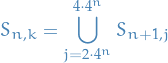
so again and
is pointwise increasing.
Let be a measure space.
Let
be nonnegative, measurable functions
s.t.
is defined
be a sequence of nonnegative measurable functions.
Then
Finite sum
Scalar multiplication
Infinte sums
Let and
be increasing sequence of simple functions converging to
,
, respectively.
Note is aslo increasing to
.
By monotone convergence theorem
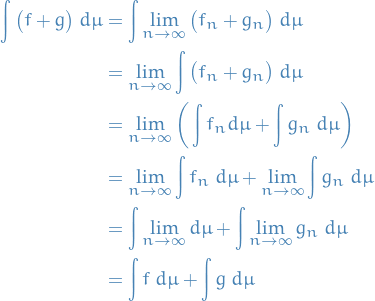
The argument is similar for products.
Finally, is an increasing sequence of nonnegative measurable functions, since sums of measurable functions is a measurable function.
Thus, by monotone convergence and the result for finite sums
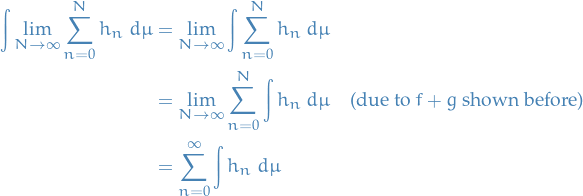
Integrals on sets
Let be a measure or probability space.
If is a sequence of disjoint measurable sets then
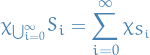
Let be a measure or probability space.
If is a simple function and
is a measurable set, then
is a simple function.
Let be a measure or probability space.
Let be a nonnegative measurable function and
.
The integral of on
is defined to be
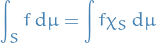
Let be a measure or probability space.
Let be a nonnegative measurable function.
If
and
are disjoint measurable sets, then
If
are disjoint measurable sets, then
Let be a measure or probability space.
If is a nonnegative measurable function, then
defined by
:
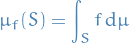
is a measure on .
If , then
defined by
:
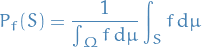
The (real) Gaussian measure on is defined as:

where denotes the Lebesgue measure.
A Gaussian probability measure can also be defined for an arbitrary Banach space as follows:
Then, we say is a Gaussian probability measure on
if and only if
is a Borel measure, i.e.

such that is a real Gaussian probability measure on
for every linear functional
, i.e.
.
Here we have used the notation , defined

where denotes the Borel measures on
.
Integrals of general functions
Let be a measure or probability space.
If is a measurable function, then the positive and negative parts are defined by
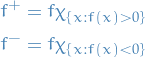
Note: and
are nonnegative.
Let be a measure or probability space.
If is a measurable function, then
and
are measurable functions.
Let be a measure or probability space.
- A nonnegative function is defined to be integrable if it is measurable and
.
- A function
is defined to be integrable if it is measurable and
is integrable.
For an integrable function , the integral of
is defined to be

On a set , the integral is defined to be

Note that , but in the actual definition of the integral, we use
.
Let be a measure or probability space.
If and
are real-valued integrable functions and
, then
(Scalar multiplication)
(Additive)
Let be a measure or probability space.
Let and
be measurable functions s.t.

If is integrable then
is integrable.
Examples
Consider
with Lebesgue measure. Is
integrable?

And
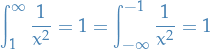
and
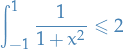
therefore

Thus, is integrable.
Lebesge dominated convergence theorem
Let be a measure or probability space.
Let be a nonnegative integrable function and let
be a sequence of (not necessarily nonnegative!) measurable functions.
Asssume and all
are real-valued.
If and
such that

and the pointwise limit

exists.
Then

That is, if there exists a "dominating function" , then we can "move" the limit into the integral.
Since and
such that
, we find
that
and
are nonnegative.
Consider
From Fatou's lemma, we have
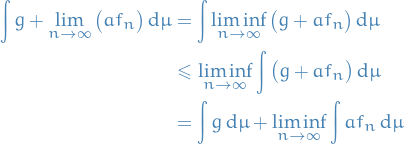
Therefore

Consider , then

(this looks very much like Fatou's lemma, but it ain't; does not necessarily have to be nonnegative as in Fatou's lemma)
Consider

Therefore,

Which implies

Since , we then have
exists and is equal to
.
Examples of failure of dominated convergence
Where dominated convergence does not work
On with Lebesgue measure, consider
![\begin{equation*}
\begin{split}
f_n &= \chi_{[n, n + \frac{1}{2}]} \\
g_n &= \arctan \big( x - n \big) + \frac{\pi}{2}
\end{split}
\end{equation*}](../../assets/latex/measure_theory_86b9124678b092e327558bdc249245b93ada07c3.png)
such that instead of
as "usual" with
.
Both of these are nonnegative sequences that converge to pointwise.
Notice there is no integrable dominating function for either of these sequences:
would require a dominating function to have infinite integral, therefore no dominating integrable function exists.
on the right, and so a dominating function would have to be above
on some interval
which would lead to infinite integral.
Thus, Lebesgue dominated convergence does not apply
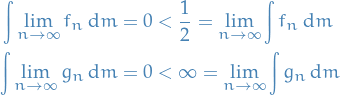
Noncummtative limits: simple case

Noncommutative limits: another one
Consider with Lebesgue measure and
![\begin{equation*}
f(x) = \frac{1}{x} \chi_{[1, \infty)} - \frac{1}{\left| x \right|} \chi_{(-\infty, -1]}
\end{equation*}](../../assets/latex/measure_theory_14635fa0f57a58ce700b3856836b8bcda95d51e0.png)
Consider and $ b > 1$ and
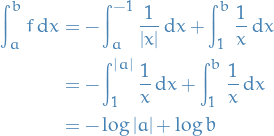
Note that , so
is not integrable.
Consider
![\begin{equation*}
\begin{split}
\lim_{N \to \infty} \int \chi_{[-N, N]} f \dd{x} &= \lim_{N \to \infty} \Big( - \log \left| - N \right| + \log N \Big) = \lim_{N \to \infty} 0 = 0 \\
\lim_{N \to \infty} \int \chi_{[-N, 2N]} f \dd{x} &= \lim_{N \to \infty} \Big( - \log N + \log 2N \Big) = \lim_{N \to \infty} \log 2 = \log 2
\end{split}
\end{equation*}](../../assets/latex/measure_theory_7503b43d535ab9bfd060eaa19b94e17bd2de244e.png)
Commutative limits
Consider
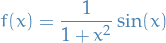
![\begin{equation*}
\lim_{N \to \infty} \lim_{M \to \infty} \int \chi_{[-M, N]} f \dd{x} = \lim_{M \to \infty} \lim_{N \to \infty} \int \chi_{[- M, N]} f \dd{x}
\end{equation*}](../../assets/latex/measure_theory_1591ddcfb29c1faa905731373db01dfdb888bb3e.png)
We know that is integrable and for all
and
,
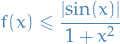
By multiple applications of LDCT
![\begin{equation*}
\begin{split}
\lim_{N \to \infty} \lim_{M \to \infty \to \infty} \int \chi_{[-M, N]} f \dd{x} &= \lim_{N \to \infty} \int \chi_{(-\infty, N]} f \dd{x} \\
&= \int \chi_{(-\infty, \infty)} f \dd{x} \\
&= \int f \dd{x} \\
&= \int \chi_{(-\infty, \infty)} f \dd{x} \\
&= \lim_{M \to \infty} \int \chi_{[-M, \infty)} f \dd{x} \\
&= \lim_{M \to \infty} \lim_{N \to \infty} \int \chi_{[-M, N]} f \dd{x}
\end{split}
\end{equation*}](../../assets/latex/measure_theory_dbd2a8cecbc43b92c2b5adc3837df5b30a770f80.png)
Showing that in this case the limits do in fact commute.
Riemann integrable functions are measurable
All Riemann integrable functions are measurable.
For any Riemann integrable function, the Riemann integral and the Lebesgue integral are equal.
Almost everywhere and Lp spaces
If is a nonnegative, measurable function, and
, then
.
For , let
![\begin{equation*}
\begin{split}
T_0 &= f^{-1} \Big( (1, \infty] \Big) \\
T_n &= f^{-1} \bigg( \bigg( \frac{1}{n + 1}, \frac{1}{n} \bigg] \bigg), \quad n \in \mathbb{Z}^+
\end{split}
\end{equation*}](../../assets/latex/measure_theory_2ceab74592bc736a2823d5c8f0aa92dafd661f57.png)
Observe the are disjoint and
![\begin{equation*}
\bigcup_{i = 1}^{\infty} T_i = f^{-1} \bigg( (0, \infty] \bigg)
\end{equation*}](../../assets/latex/measure_theory_93b55f6349b02c3782503473a25b3f7b47350719.png)
Suppose that . This implies that
on a set of positive measure, i.e.
![\begin{equation*}
\mu \bigg( f^{-1} \Big( (0, \infty] \Big) \bigg) > 0
\end{equation*}](../../assets/latex/measure_theory_e85507015506242700deeda1834343a94f438944.png)
but this implies that
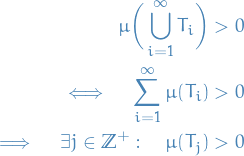
Thus,
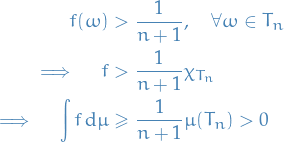
which is a contradiction, hence .
Let and
be integrable.

is the set of all equivalence classes of integrable functions wrt. the equivalence relation given by a.e. equality, i.e.

If is an integrable function, the
norm is
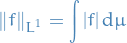
If and
, the integral and norm are defined to be
![\begin{equation*}
\begin{split}
\int [f] \dd{\mu} &= \int g \dd{\mu} \\
\norm{[f]}_{L^1} &= \norm{g}_{L^1}
\end{split}
\end{equation*}](../../assets/latex/measure_theory_6917d223cd066748131b36bb5557b619ee598345.png)
If , then
, and
![\begin{equation*}
\norm{[f - g]}_{L^1} \le \norm{f - g}_{L^1} + \norm{[h - g]}_{L^1}
\end{equation*}](../../assets/latex/measure_theory_280aa5fd4f0f61951e29cf2fba4c67eb4af32159.png)
is a real vector space with addition and scalar multiplication given pointwise almost everywhere.
Functions taking on on a set of zero measure are fine!
These functions are still the almost everywhere equal to some integrable function (even those these infinite-valued functions are integrable), hence these are in .
Let be a Cauchy sequence. Since the
are integrable, we may assume we choose
valued representatives.
For , let
be such that for
,
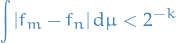
and .
Thus,

and

Thus, is finite almost everywhere. Thus, this series is infinite on a set of measure zero, so we may assume the representatives
are zero there and the sum is finite at each
.
Thus, converges everywhere.
Let
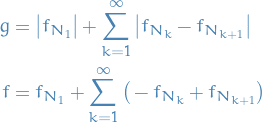
(observe that the last part is just rewriting the ).
By monotone convergence theorem
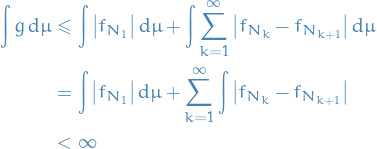
Observe that pointwise
Applications to Probability
Notation
is a probability space
- Random variable
is a measurable function
denotes the Borel sigma-algebra on
denotes the probability distribution measure for
be a sequence of random events
be a sequence of finitely many random events
Probability and cumulative distributions
An elementary event is an element of .
A random event is an element of
A random variable is a measurable function from to
.
Let
be a measure space and
be a measurable space
be a measurable function
Then we say that the push-forward of by
is defined

The probability distribution measure of , denoted
, is defined

Equivalently, it's the push-forward of by
:

In certain circles not including measure-theorists (existence of such circles is trivial), you might hear talks about "probability distributions". Usually what is meant by this is for some random variable
.
That is, a "distribution of " usually means that there is some probability space
in which
is a random variable, i.e.
and the "distribution of
" is the corresponding probability distribution measure!
Confusingly enough, they will often talk about " distribution of
", in which case
is NOT a probability measure, but denotes a probability distribution measure of the random variable.
The cumulative distribution function of , denoted
, is defined by
![\begin{equation*}
\forall x \in \mathbb{R}, \quad F_X(x) = P(X \le x) = \rho_X \big( (- \infty, x] \big)
\end{equation*}](../../assets/latex/measure_theory_1135df16d8d98e78eda428fdded8af11029fc26f.png)
where is the probability distribution measure of
.
The probability distribution measure is a probability measure on the Borel sets
.

If is a disjoint sequence of sets in
, then
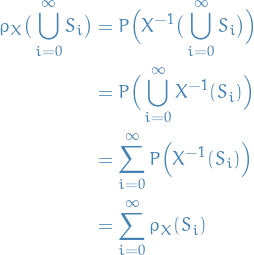
so satisfies countable additivity and is a measure.
Finally,

so is a probability measure.
is increasing
and
is right continuous (i.e. continuous from the right)
If
, then
Consider the limit as
. Let
so
Then,
which, since
is increasing implies
Let
and
. Let
The
are nested, and similarily
are nested.
Thus, given
, there exists
such that
Let
so
Radon-Nikodym derivatives and expectations
Let
be a rv.
its probability distribution measure
its cumulative distribution function
a Borel measureable function
The following are equivalent:
is a Radon-Nikodym derivative for
wrt.
(the Lebesgue measure but restricted to Borel measurable sets)
(2) and (3) are immediately equivalent:
![\begin{equation*}
\rho_X \Big( (- \infty, x] \Big) = F_X(x) = \int_{-\infty}^{x} f(s) \dd{s} = \int_{-\infty}^{x} f \dd{m}
\end{equation*}](../../assets/latex/measure_theory_6826f1b8d841609a361882c3b6b70bc5ea1174ef.png)
iff (2) or (3) holds when considering only sets of the form .
This statement is also equivalent to (1).
Thus (1) is equivalent to (2) or (3) restricted to sets of the form .
However, sets of the form generate
, so from the Carathéodory extension theorem this gives
.
To prove more rigorously, let
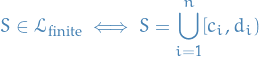
for s.t.
and none of these intervals overlap. That is all finite unions of left-closed, right-open, disjoint intervals.
Also let
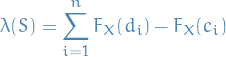
Observe that
![\begin{equation*}
\lambda(S) = \sum_{i=1}^{n} \bigg[ \int_{-\infty}^{d_i} f(x) \dd{x} - \int_{-\infty}^{c_i} f(x) \dd{x} \bigg] = \sum_{i=1}^{n} \int_{c_i}^{d_i} f(x) \dd{x} = \int_S f \dd{m}
\end{equation*}](../../assets/latex/measure_theory_a1afc8f6f2c8e420ab88be7447f2e38b913fb942.png)
and that
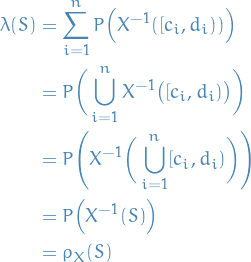
One can show that is a premeasure space. Therefore, by the Carathéodory extension theorem, there is a measure
on
s.t.

Furthermore, since ,
is unique! But both the measures
and
satisfy these properties, thus

which is the definition of being a Radon-Nikodym derivative of
wrt. Lebesgue measure restricted to the Borel σ-algebra, as wanted.
A function is a probability density function for
if
is a Radon-Nikodym derivative of the probability distribution measure
, wrt. Lebesgue measure restricted to Borel sets, i.e.
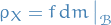
Expectation via distributions
Expectation of a random variable is
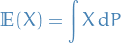
If is a nonnegative function that is
measurable, then
![\begin{equation*}
\mathbb{E} \big[ g(X) \big] = \int g(s) \dd{\rho_X(s)}
\end{equation*}](../../assets/latex/measure_theory_58b872956e73f042002d8b23eb8c33aa0d3cde55.png)
If is the characterstic function, then, if
,
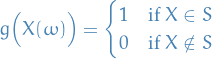
so

Multiplying by constants and summing over different characteristic functions, we get the result to be true for any simple function.
Given a nonnegative function , let
be an increasing sequence of simple functions converging pointwise to
.
Note is the increasing limit of
. By two applications of Monotone Convergence
![\begin{equation*}
\begin{split}
\mathbb{E} \big[ g(X) \big] &= \int g(X) \dd{P}\\
&= \int \lim_{n \to \infty} g_n(X) \dd{P} \quad\\
&= \lim_{n \to \infty} \int g_n(X) \dd{P} \quad \text{(by MC)} \\
&= \lim_{n \to \infty} \int g_n \dd{\rho_X} \quad \text{(by above)}\\
&= \int g \dd{\rho_X} \quad \text{(by MC)}
\end{split}
\end{equation*}](../../assets/latex/measure_theory_011ad9343b3c73146cd5357e27623d0048277ef0.png)
This techinque, of going from characterstic function → simple functions → general functions, is used heavily, not just in probability theory.
Independent events & Borel-Cantelli theorem
A collection of random events are independent events if for every finite collection of distinct indices
,
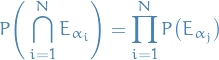
A random event occurs at
if
.
The probability that the event occurs is .
If are independent then
are also independent.
Prove that are independent.
Consider , we want to prove

RHS can be written
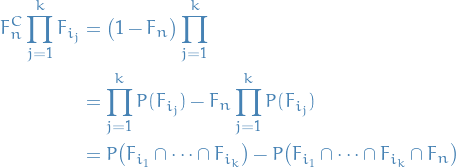
which is equal to LHS above, and implies that the complement is indeed independent.
The condition that infinitively many of the events occurs at is
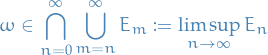
This is equivalent to

where we have converted the and
.
Furthermore, is itself a random event.
If
then probability of infinitely many of the events occuring is 0, i.e.
If the
are independent and
, then probability of infinitely many of the events occuring is 1, i.e.
Suppose
.
Suppose
are now independent and that
. Fix
. Then
Chebyshev's inequality
Let be a probability space.
If is a random variable with mean
and variance
, then

Let

Then everywhere, so
![\begin{equation*}
\sigma^2 = \mathbb{E} \big[ \big( X - \mu \big)^2 \big] \ge \lambda^2 \mathbb{E} \big[ \chi_E \big] = \lambda^2 P(E)
\end{equation*}](../../assets/latex/measure_theory_afe5a26fe68a2dc369c6842791542f5d9f5bcae3.png)
Hence,

Independent random variables
Let
be a probability space.
A collection of σ-algebras , where
for all
, is independent if for every collection of events
s.t
for all
, then
is a set of independent events.
A collection of random variables is independent if the collection of σ-algebras they generate is independent.
A sequence of random variables is independent and identically distributed (i.i.d) if they are independent variables and for
we have

where is the cumulative distribution function for
.
Let and
be independent.
- We have
- If
or
then
If
and
, then
- If
Furthermore, if
and
, then
Consider
- first nonnegative functions
- subcase
Since is nonnegative
![\begin{equation*}
0 = \mathbb{E} \big[ X \big] = \int X \dd{P} \implies X \overset{\text{a.s.}}{=} 0
\end{equation*}](../../assets/latex/measure_theory_3adfb341d10c51cb63db312ac51f907340ca7087.png)
Thus, so
.
Now consider the subcase where and
.
Let and
be the σ-algebras generated by
and
.
Observe that and
are measure spaces. Let
be an increasing sequence of simple functions that are measurable wrt.
and similarily
simple increasing to
and
measurable.
As simple functions, these can be written as
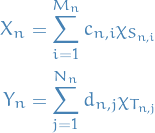
Then,
![\begin{equation*}
\begin{split}
\mathbb{E} \big[ X_n Y_n \big] &= \int \sum_{i=1}^{M_n} \sum_{j=1}^{N_n} c_{n, i} d_{n, j} \chi_{S_{n, i}} \chi_{T_{n, j}} \dd{P} \\
&= \sum_{i=1}^{M_n} \sum_{j=1}^{N_n} c_{n, i} d_{n, j} P \Big( S_{n, i} \cap T_{n, j} \Big) \\
&= \sum_{i=1}^{M_n} \sum_{j=1}^{N_n} c_{n, i} d_{n, j} P \big( S_{n, i} \big) P \big( T_{n, j} \big) \\
&= \bigg( \sum_{i=1}^{M_n} c_{n, i} P \big( S_{n, i} \big) \bigg) \bigg( \sum_{j=1}^{N_n} d_{n, j} P\big(T_{n, j} \big) \bigg) \\
&= \mathbb{E} \big[ X_n \big] \mathbb{E} \big[ Y_n \big]
\end{split}
\end{equation*}](../../assets/latex/measure_theory_a8103249f0b23d4dc0542716a5f5c9d173d60a25.png)
Since increases to
, by MCT
![\begin{equation*}
\mathbb{E} \big[ XY \big] = \mathbb{E} \big[ X \big] \mathbb{E} \big[ Y \big]
\end{equation*}](../../assets/latex/measure_theory_127c9fbe3926cc0ee673512d405f56dccef62e67.png)
Dividing into positive & negative parts & summing gives .
Strong Law of Large numbers
Notation
are i.i.d. random variables, and we will assume
Stuff
Let be a probability space and
be a sequence of i.i.d. random variables with
![\begin{equation*}
\mu := \mathbb{E}[X_i] < \infty \quad \text{and} \quad \sigma^2 := \text{Var}(X_i) < \infty
\end{equation*}](../../assets/latex/measure_theory_b5a2be16754e0eac4426741ec560e711c0165643.png)
Then the sequence of random variables converges almost surely to
, i.e.
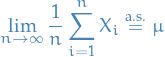
This is equivalent to occuring with probability 0, and this is the approach we will take.
First consider .
For and
, let
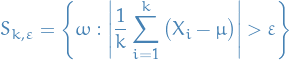
and
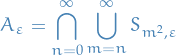
Since are i.i.d. we have
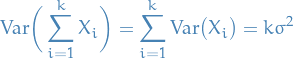
and since variance rescales quadratically,
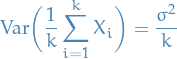
Using Chebyshev's inequality

Observe then that with , we have

And so by Borel-Cantelli, since this is a sequence of independent random variables, we have
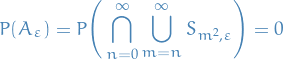
In particular, for any , there are almost surely only finitely many
with
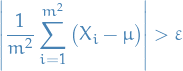
Step: showing that we can do this for any .
Consider . Observe that by countable subadditivity,
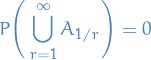
Now let , which occurs almost surely from the above. For any
, let

Since , there are only finitely many
s.t.
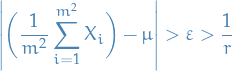
as found earlier (the parenthesis are indeed different here, compared to before). Therefore

is arbitrary, so this is true for all
. Hence,

This proves that there is a subsequential limit almost surely.
Step: subsequential limit to "sequential" limit.
Given , let
be such that
. Since
are nonnegative
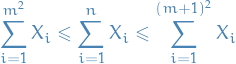
and therefore

and since ,

Since the first and the last expressions converge to ,t by the squeeze theorem we have
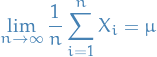
Step: Relaxing nonnegativity assumption on .
Suppose is not necessarily nonnegative. Since, by assumption,
has finite expectation,
is integrable. Therefore we know that the positive and negative parts of
, denoted
, are also integrable. Therefore we can compute the expectations

Similarily, we have that the variance of is finite, which allows us to the apply the result we found for
being nonnegative to both
and
:

Let be the set where the mean of the positive / negative part converges. Since
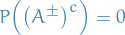
(since otherwise the limit would not converge almost surely). We then have
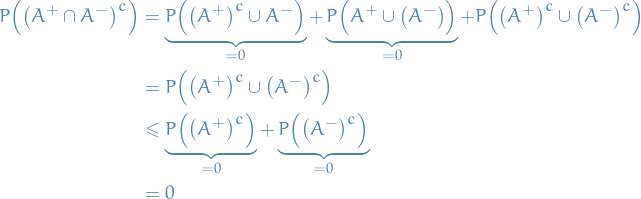
Thus, almost surely, , and on this we have convergence, so
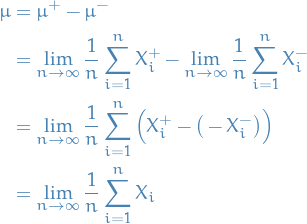
Concluding our proof.
Ergodic Theory
Let be a measure-preserving transformation on a measure space
with
, i.e. it's a probability space.
Then is ergodic if for every
we have

Bochner integrable
The Bochner integral is a notion of integrability on Banach spaces, and is defined in very much the same way as integrability wrt. Lebesgue-measure.
Let be a measure space and
a Banach space.
A simple function is defined similarily as before, but now taking values on a Banach space instead. That is,

with the integral
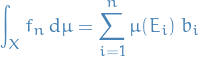
A measurable function is said to be Bochner integrable if there exists a sequence of integrable simple functions
such that

where the integral on the LHS is an ordinary Lebesgue integral.
If this is the case, then the Bochner integral is defined
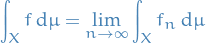
It can indeed be shown that a function is Bochner integrable if and only if
, the
Bochner space, defined similarily as L1-space for functions but with the absolute value replaced by the
.
Concentration inequalities
Stochastic processes
Let
be a filtration
be an
martingale
be an
stopping time
such that one of the following holds:
such that
and there exists a constatn
s.t. for all
,
almost surely on the even that
.
such that
almost surely for all
Then is a.s. well-defined and
.
Furthermore, when is supersub-martingale rather than a martingale, then equality is replaced with lessgreather-than, respectively.
Let be a supermartingale with
a.s. for all
.
Then for any
![\begin{equation*}
\mathbb{P} \bigg( \sup_{t \in \mathbb{N}} X_t \ge \varepsilon \bigg) \le \frac{\mathbb{E}[X_0]}{\varepsilon}
\end{equation*}](../../assets/latex/measure_theory_84492b275ebda66c6570d1bd84ca669f463b7733.png)
Let be the event that
and
, where we assume
so that
if
for all
.
Clearly is a stopping time and
. Then by Doob's optional stopping theorem and an elementary calculation
![\begin{equation*}
\begin{split}
\mathbb{E} [X_0] & \ge \mathbb{E}[X_{\tau}] \\
&\ge \mathbb{E} \big[ X_{\tau} \1 \left\{ \tau \le n \right\} \big] \\
&\ge \mathbb{E} \big[ \varepsilon \1 \left\{ \tau \le n \right\} \big] \\
&= \varepsilon \mathbb{P} (\tau \le n) = \varepsilon \mathbb{P} (A_n)
\end{split}
\end{equation*}](../../assets/latex/measure_theory_94b8b81bf7fe44c285cfefc52fe78eb1118bd0f2.png)
Course: Advanced Probability
Notation
is used as a binary operation which takes minimum of the two arguments
is used as a binary operation which takes maxmium of the two arguments
Lecture 1
Notation
denotes a measurable space (with a measure
it becomes a measure space)
denotes the set of measurable functions wrt.
and non-negative measurable functions
We write
Stuff
Let be a measure space.
Then there exists a unique s.t.
for all
Linearity
for all
with
.
-
for
pointwise.
There exists a unique measure on
called the product measure

Let . For
define

Then is
. Hence, we can define
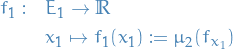
Then is
and

where is the product measure.
Applying the above in both directions, we have

with

and

Conclusion:

Lecture 2: conditional expectation
Notation
a probability space, i.e.
denotes rv, i.e.
is
and integrable, with expectation
Also write
or, as we're used to,
instead of
Stuff
Let with
.
Then
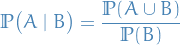
is called the conditional probability of given
.
Similarily, we define
![\begin{equation*}
\mathbb{E} \big[ X \mid B \big] = \frac{\mathbb{E}[X 1_B]}{\mathbb{P}(B)}
\end{equation*}](../../assets/latex/measure_theory_1e9e33511ea4e04d0d2b93b6e59baf140240ac27.png)
to be the conditional expectation of given
.
- Quite restrictive since we require probability of
to be non-zero
- Goal: improve prediction for
if additional "information" is available
- "Information" is modelled by a sigma-algebra
- "Information" is modelled by a sigma-algebra
Let be a sequence of disjoint events, whose union is
. Set

For any integrable random variable , we can define
![\begin{equation*}
Y = \sum_{n \in \mathbb{N}}^{} \mathbb{E} \big[ X \mid B_n \big] 1_{B_n}
\end{equation*}](../../assets/latex/measure_theory_df0b9f2767d97d3a5e3b87e10ea91d7c6a6b2dfa.png)
where we set
![\begin{equation*}
\mathbb{E} \big[ X \mid B_n \big] =
\begin{cases}
\frac{\mathbb{E} [ X 1_{B_n}]}{\mathbb{P}(B_n)} & \text{if } \mathbb{P}(B_n) > 0 \\
0 & \text{if } \mathbb{P}(B_n) = 0
\end{cases}
\end{equation*}](../../assets/latex/measure_theory_cb9ab6ebd3778592daaf856ee81c1ce9ae052dd8.png)
Notice that
in (discrete) definition of conditional expectation is
Let
, then
because
, and each of these sets are measurable Notice that this is simply the union of intersections
which is just
But this is just
since
! That is,
Which means we end up with
which is union of
sets and so
is
random variable.
is integrable and
This is easily seen from
since
and
is integrable.
There's an issue with the (discrete) definition of conditional expectation though. Example:
,
and
be the Lebesgue measure
Consider the case
- Then consider
is a rv.
Then let
Then
Issue: if
has an absolutely continuous distribution, e.g.
, i.e.
then the set we're summing over
is the empty set!
- This motivates the more general defintion which comes next!
Let with
is a σ-algebra.
A random variable is called (a version of) the conditional expectation of
given by
if
is
And
So we write
can be replaced by
throughout
- If
with
it suffices to check for all
If
with
a rv., then
is
by condition (1) in def of conditional expectation, so it's of the form
for some function
; therefore it's common to define
Let with
a sigma-algebra.
Then
exists
- Any two versions of
coincide
- Let
be as in conditional expectation and let
satisfy the conditions in the same def for some
with
almost surely.
- Let
with
(in
because both
are
)
Then
since
. The first equality is due to condition (2) in def of cond. expectation.
implies, by def of
, that
- If
, a similar argument shows that
(using
and
)
- The reason why we did the inequality first is because we'll need that later on.
- Let
- We're going to do this by orthogonal projection in
.
Assume
. Since
is a complete subspace of
, so such
has an orthogonal projection
on
, i.e.
Choosing
for some
, we get
so
satisfies (1) and (2) in def of cond expectation, from equation above. But this is assuming
which is not strict enough for the case when
! So we gotta do some more work.
Assume
. Then
and
for some
. By Step 1, we know that
and
a.s. (by proof of (2) above). Further, let
with
which is just the set where the sequence is increasing. Then
is
and by MCT we get
Then, letting
,
so
a.s. (and thus
) and
satisfies the condtions in def of cond expectation
For general
, apply Step 2 on
and
to obtain
and
. Then
satisfies the condtions in def of cond expectation.
Let , i.e. integrable random variable, and let
be a σ-algebra.
We have the following properties:
- If
is
, then
- If
is independent of
, then
- If
, then
.
For
and any integrable random variable
, we have
Let be a sequence of random variables. Suppose further
a.s., then
a.s., for some
random variable
.
(conditional MCT) By MCT, we therefore have
which implies that
This is basically the conditional MCT:
(conditional Fatou's lemma)
(conditional Dominated convergence) If
and
for all
, almost surely, for some integrable variable
, then
(conditional Jensen's inequality) If
is convex, then
In particular, for
, we have
where we used Jensen's inequality for the inequality. Thus we have
For any σ-algebra , the rv.
is
and satisfies, for all
,
![\begin{equation*}
\mathbb{E} \big[ Y 1_A \big] = \mathbb{E} \big[ \mathbb{E}[X \mid \mathcal{G}] 1_A \big] = \mathbb{E}[X 1_A]
\end{equation*}](../../assets/latex/measure_theory_6144fe4dcd295a073ccaab093d7bd52be3094166.png)
(Tower property)
"Take out what is known": if
is bounded and
, then
since
is then
and
(actually, you need to first consider
for some
, and then extend to all measurable functions
using simple functions as usual)
If
is independent of
, then
Because suppose
and
, then
The set of such intersections
is a π-system generating
, so the desired formula follows from [SOME PROPOSITION].
From defintion of conditional expectation we know that
And since
, we must also have
Let
. Observe that
since
and both
and
are
, thus the their preimages must be in
and
Moreover, from the definition of
, we know that
which implies
i.e.
[TODO]
being independent of
means that
Let
since
is
. Then
by def of expectation. But
- Proven in proof:1.4.-lec-existence-and-uniqueness-of-conditional-expectation
If we can show that properties (1) and (2) from def:conditional-expectation is satisfied by
, then by (2) we get LHS immediately. Measurability follows from linear combination of measurables being measurable. And observe that for all
by the fact that
and
are both
.
Let . Then the set of random variables
of the form
![\begin{equation*}
Y = \mathbb{E}[ X \mid \mathcal{G}]
\end{equation*}](../../assets/latex/measure_theory_934170d3276903877c58696d9c5f1d6786180970.png)
where is a σ-algebra is uniformly integrable.
Given , we can find
so that
![\begin{equation*}
A \in \mathcal{F} : \mathbb{P}(A) \le \delta \quad \implies \quad \mathbb{E} [ \left| X \right| 1_A ] \le \varepsilon
\end{equation*}](../../assets/latex/measure_theory_c63034d14d67202fc9f811fdf605ed168a34abd2.png)
Then choose so that
![\begin{equation*}
\mathbb{E}[|X|] \le \lambda \delta
\end{equation*}](../../assets/latex/measure_theory_f82abdc8f13a080efa56b7c9d0d4a8bc4fa42285.png)
Suppose , then
. In particular,
![\begin{equation*}
\mathbb{E}[\left| Y \right|] \le \mathbb{E}[\left| X \right|]
\end{equation*}](../../assets/latex/measure_theory_fc6c3f166386273284e03ff2da803ef06fc4712a.png)
so, by Markov's inequality, we have
![\begin{equation*}
\mathbb{P} \big( \left| Y \right| \ge \lambda \big) \le \frac{\mathbb{E}[\left| Y \right|]}{\lambda} \le \delta
\end{equation*}](../../assets/latex/measure_theory_02028dcf4735fc5a1563da8067bfa3872f190eb5.png)
Then
![\begin{equation*}
\mathbb{E} \big[ \left| Y \right| 1_{\left| Y \right| \ge \lambda} \big] \le \mathbb{E} \big[ \left| X \right| 1_{\left| Y \right| \ge \lambda} \big] \le \varepsilon
\end{equation*}](../../assets/latex/measure_theory_cd993aa8c7e21c90a3b5b49436029e37bfe715a3.png)
Since our choice of was independent of
, we have our proof for any σ-algebra
.
Martingales in discrete time
Let be a probability space.
A filtration on this space is a sequence of σ-algebras such that,

We also define

Then .
A random process (in discrete time) is a sequence of random variables .
Let be a random process (discrete time).
Then we define the natural filtration of to be
, given by

Then models what we know about
by time
.
We say is adapted to
if
is
for all
.
This is equivalent to requiring that for all
.
Let
be a filtration
is
if
is
for each
.
We say a random process is integrable if is an integrable random variable for all
.
A martingale is an adapted random process (discrete time) such that
![\begin{equation*}
\mathbb{E} \big[ X_{n + 1} \mid \mathcal{F}_n \big] \overset{\text{a.s.}}{=} X_n, \quad \forall n \ge 0
\end{equation*}](../../assets/latex/measure_theory_deae7c487620e3d5e90aa8c282b77d7c9adadbc7.png)
If instead
![\begin{equation*}
\mathbb{E} \big[ X_{n + 1} \mid \mathcal{F}_n \big] \overset{\text{a.s.}}{\le} X_n, \quad \forall n \ge 0
\end{equation*}](../../assets/latex/measure_theory_374dfc453829912cf00bb05259cacf1e7025120c.png)
we say is a supermartingale.
And if instead
![\begin{equation*}
\mathbb{E} \big[ X_{n + 1} \mid \mathcal{F}_n \big] \overset{\text{a.s.}}{\ge} X_n, \quad \forall n \ge 0
\end{equation*}](../../assets/latex/measure_theory_a53395fc7afac54371a797ed90d1f62ca8abf65a.png)
we say is a submartingale.
Every process which is martingale wrt. a given filtration is also martingale wrt. its natural filtration.
We say a random variable

is a stopping time if for all
.
For a stopping time , we set

Let and
be stopping times and let
be an adapted process. Then
is a stopping time
is a σ-algebra
- If
, then
is an
random variable
is adapted
- If
is integrable, then
is integrable.
Let
denote a probability space
be a filtration
adapted to
Note that
And, since
and
are a stopping times,
which also implies
, since a σ-algebra is closed under complements
- Similarily for
- σ-algebra is closed under finite intersections and unions
Hence
Importantly this holds for all
, and so
i.e.
is a stopping time.
Recall
which can equivalently be written
Problem sheets
PS1
1.1
Let and let
be a σ-algebra. Then
![\begin{equation*}
\mathbb{E}[X + Y \mid \mathcal{G}] \overset{\text{a.s.}}{=} \mathbb{E}[X \mid \mathcal{G}] + \mathbb{E}[Y \mid \mathcal{G}]
\end{equation*}](../../assets/latex/measure_theory_8cf470a61f13927948574342066439e7948c7ba2.png)
First of, is
because each
and
are
and we know that linear combinations of measurables are measurable.
Second,
![\begin{equation*}
\begin{split}
\mathbb{E} \big[ (\mathbb{E}[X \mid \mathcal{G}] + \mathbb{E}[X \mid \mathcal{G}]) 1_A \big] &= \mathbb{E} \big[ \mathbb{E}[X \mid \mathcal{G}] 1_A + \mathbb{E}[Y \mid \mathcal{G}] 1_A \big] \\
&= \mathbb{E} \big[ \mathbb{E}[X \mid \mathcal{G}] 1_A \big] + \mathbb{E} \big[ \mathbb{E}[Y \mid \mathcal{G}] 1_A \big] \\
& \overset{\text{a.s.}}{=} \mathbb{E} [X 1_A] + \mathbb{E}[Y 1_A] \\
&= \mathbb{E} \big[ X 1_A + Y 1_A \big] \\
&= \mathbb{E} \big[ (X + Y) 1_A \big]
\end{split}
\end{equation*}](../../assets/latex/measure_theory_75db366a205975b526e4b88cb86638110241baf9.png)
1.2
Let
be a non-negative rv.
be a version of
Then

i.e.

Further,

Course: Advanced Financial Models
Notation
Lecture 2
If is
, then
a.s.
Conversely, if a.s. then
s.t

i.e. everything "interesting" happens in the intersection of and
If then
![\begin{equation*}
\begin{split}
\mathbb{P}(A \mid \mathcal{G}) &= \mathbb{E} \big[ 1_A \mid \mathcal{G} \big] \\
&= 1_A \in \left\{ 0, 1 \right\}
\end{split}
\end{equation*}](../../assets/latex/measure_theory_4ee4b85118a52b5e2ac8e67f3c90e080b7396d2e.png)
since is
.
Suppose where
.
Note tthat
![\begin{equation*}
\begin{split}
\mathbb{E} \big[ (1_A - 1_{A'})^2 \big] &= \mathbb{P}(A) + \mathbb{P}(A') - 2 \mathbb{P}(A \cup A') \\
&= 2 \big( \mathbb{P}(A) - \mathbb{P}(A \cap A') \big) \\
&= 0
\end{split}
\end{equation*}](../../assets/latex/measure_theory_399fc3424aa36443a24a97e2ce82bb6edd7dae0a.png)
where in the last equality we've used
![\begin{equation*}
\begin{split}
\mathbb{P}(A \cap A') &= \mathbb{E} \Big[ \mathbb{E} \big[ 1_A - 1_{A'} \mid \mathcal{G} \big] \Big] \\
&= \mathbb{E} \big[ 1_{A'} \mathbb{P}(A \mid \mathcal{G}) \big] \\
&= \mathbb{P}(A') \\
&= \mathbb{P}(A)
\end{split}
\end{equation*}](../../assets/latex/measure_theory_a9c8baa9226f515ae88d9255c4fcf3e574d1228a.png)