Gradient Boosting
Table of Contents
Gradient boosting
Overview
Gradient boosting is a technique for regression and classification problems, which produces a prediction model in the form of an ensemble of weak prediction models, typically prediction trees. It builds the model in a stage-wise fashion like other boosting methods do, and generalizes them by allowing optimization of an arbitrary differentiable loss function.
Motivation
Consider a least-squares regression setting, where the goal is to "teach" a model to predict values in the form
by minimizing the mean squared error
, averaged over some training set of actualy values of the output variable
.
At each stage , of gradient boosting, it may be assumed that there is a some imperfect model
. The gradient boosing algorithm improves on
by constructing a new model that adds an estimator
to provide a better model:
.
To find , the gradient boosting solution starts with the observation that a perfect
would imply

i.e. that adding the extra predictor will make up for all the mistakes our previous model
did, or equivalently

Therefore, gradient boosting will fit to the residual
. Like in other boosting variants, each
learns to correct its predecessor
.
The generalization to other loss-functions than the least-squares, comes from the observation that for a given model are the negative gradients (wrt.
) of the squared error loss function, hence for any loss function
we'll simply fit to the negative gradient
.
Algorithm
Input: training set , a differentiable loss function
, number of iterations
.
Initialize model with a constant value:
For
to
:
- Compute so-called psuedo-residuals :
- Fit a base learner (e.g. tree)
to psuedo-residuals, i.e. train it using the training set
- Compute multiplier
sby solving the following one-dimension optimization problem
- Update the model:
- Output
Gradient Tree Boosting
- Uses decision trees of fixed size as base learners
Modified gradient boosting method ("TreeBoost")
- Generic gradient boosting at the m-th step would fit a decision tree
to psuedo-residuals
- Let
be the number of its leaves
Tree partitions input space into
disjoint regions
and predicts a constant value in each region, i.e.
where
is the value predicted in the region
.
In the case of usual CART trees, where the trees are fitted using least-squares loss, and so the coefficient for the region
is equal to just the value of the output variable, averaged over all training instances in
.
Continuing with generic gradient boosting, the model is:
The modified gradient tree boosting ("TreeBoost") algorithm is then to instead choose a separate optimal value for each of the tree's regions, instead of a single
for whole tree:
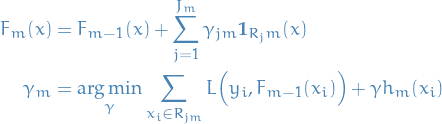
where we've simply absorbed into
.
Terminology
- Out-of-bag error / estimate is a method for measuring prediction error of methods utilizing bootstrap aggregating (bagging) to sub-sample data used for training.
OOB is the mean prediction error on each training sample
, using only the trees that did not have
in their bootstrap sample
Regularization
- Number of trees: Large number of trees can overfit
Shrinkage: use a modified update rule:
where
is the learnin rate.
- Subsampling is when at each iteration of the algorithm, a base learner should be fit on a subsample of the training set drawn at random without replacement. Sumsample size
is the fraction of training set to use; if
then algorithm is deterministic and identitical to TreeBoost
- Limit number of observations in trees' terminal nodes: in the tree building process by ignoring any split that lead to nodes containing fewer than this number of training set instances. Helps reduce variance in predictions at leaves.
- Penalize complexity of tree: model complexity can be defined as proportional number of leaves in the learned trees. Joint optimization of loss and model complexity corresponds to a post-pruning algorithm.
- Regularization (e.g.
,
) on leaf nodes