Hidden Markov Models
Table of Contents
Model
Hidden Markov Model (HHM) is a probability model used to model latent
states ( ) based on observations which depends on the current
state of the system. That is; at some step
the system is in some
"internal" / unobservable / latent state
. We then observe an
output
which depends on the current state,
, the system is in.
Derivation
- Hidden states/rvs
i.e. a discrete set of states
- Observed rvs
is the set of all possible values that
can take, e.g. discrete,
Then,

The reason why we use here is that elements
of
, can potentially be multi-dimensional, e.g.
,
then
represents a vector of observed rvs, not just
a single observed rv.
Parameters
- Transition probabilities/matrix:
- Emission probabilites:
for
is then a prob. dist. on
- Initial dist:
The difference between and
, e.g. lower- and uppercase notation
is that
represents the distribution of the rv
, while
is the actual rv, and so
means the case where the rv
takes on the value
. The notation
for some arbitrary
is then talking about a discrete distribution.
Using the substitutions from above, the join distribution is the following:

The emission prob. dist for the different values of the hidden states can be basically any prob. dist.
The plot below shows a problem where HMM might be a good application, where
we have the states in
blue
and observations in red
with
emission probability .
Forward-backward algorithm / alpha-beta recursion
Example of a dynamic programming algorithm. This provides us with a
way of efficiently estimating the probability of the system
being in some state at the
step given all
observations we've seen,
, i.e.
.
This does not provide us with a way to calculate the probability of a sequence of states, given the observations we've seen. For that we have to have a look at the Viterbi algorithm.
Notation
i.e. the sequence of observations made, not the rv representing some
represents the sequence of observations from the
observation to the
observation
Algorithm
We make the assumptions that we know ,
and
.
Our goal of the entire algorithm is to compute .
- Forward algorithm
- computes
for all
- Backward algorithm
- computes
for all
We can write our goal in the following way:

Which means that if we can solve the forward and backward algorithms
described above, we can compute .
What we're actually saying here is:
- Given that we know the value of the rv.
the sequence of observations
does not depend on the observations prior to the
observation.
- Observations prior to the
observation, does not depend on the observations in the future
Forward algorithm
Our goal is to compute .
We start out with the following:
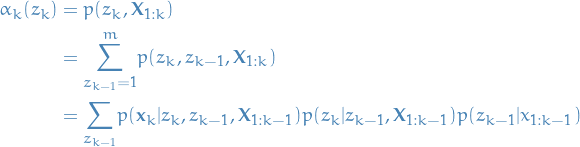
In words: probability of observing given that we have observed
the sequence
and we're in state
marginalized / summed over
the possible previous states
.
But since the observation at the step,
, is independent of
given that we know
. And so we have:

And the state,
, is independent of the previous observations
given that we know
. And so further we have:

Thus, we end up with:
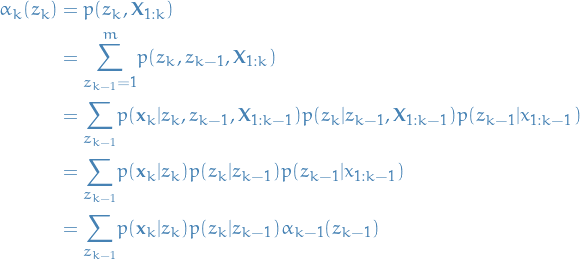
And if you go back to our assumptions you see that we have assumed
that we know the emission probabilities and
the transition probabilities
. And thus we only have
one unknown in our equation.
Tracking this recursive relationship all the way back to the ,
we end up with zero unknowns, since we also assumed to know the
initial probability
.
So just to conclude:
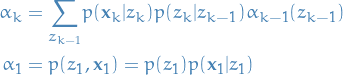
provides us with the enough info to compute in a recursive
manner.
Time complexity
- For each step
we have to sum over the
possible values for the previous state
, and so this is of time complexity
- But we have to do the step above for every possible value of
, thus we have an additional
- Finally, we gotta do
of these steps, and so we have the two steps above with total time complexity
being computed
times, leading us the time complexity of the Forward algorithm to be
This is cool as it is linear in the number of steps, !
Backward algorithm
Our goal is to compute for all
.
And again we try to deduce some recursive algorithm.
We start out with:

Then if we simply define some auxilary variable to
be

Aaaand we got ourselves a nice recursive relationship once again!
Again, by assumption, we know what and
is.
And the final step is as follows
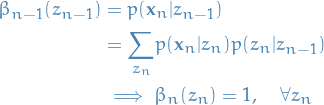
Thus, we conclude the Backward algorithm with the following:

Time complexity
Again we have the same reasoning as for the forward algorithm time complexity
and end up with time complexity of .
Implementation
Throughout this implementation we will be validating against this wikipedia example.
First we gotta turn those data representations into structures which our implementation can work with:
wiki_emission_probs = np.array([[0.9, 0.1], [0.2, 0.8]]) wiki_initial_dist = np.array([[0.5, 0.5]]) wiki_observations = np.array([0, 0, 1, 0, 0]) wiki_transition_probs = np.array([[0.7, 0.3], [0.3, 0.7]]) S_wiki = np.arange(2) T_wiki = wiki_transition_probs X_wiki = wiki_observations epsilon_wiki = lambda z, x: wiki_emission_probs[z][x] pi_wiki = np.array([0.5, 0.5])
Forward
cnt = 0 def forward(pi, X, S, epsilon, T): n_obs = len(X) n_states = len(S) alpha = np.zeros((n_obs + 1, n_states)) alpha[0] = pi for k_minus_one, x in enumerate(X): # readjust the index of alpha since # we're including pi too k = k_minus_one + 1 alpha_k = alpha[k] for z_k in S: alpha_k[z_k] = sum( # p(z_k | z_{k-1}) * p(x_k | z_k) * alpha_{k-1}(z_{k-1}) T[z, z_k] * epsilon(z_k, x) * alpha[k - 1][z] for z in S ) # gotta normalize to get probab p(z_k, x_{1:k}) alpha[k] = alpha_k / alpha_k.sum() return alpha forward(pi_wiki, X_wiki, S_wiki, epsilon_wiki, T_wiki)
array([[ 0.5 , 0.5 ], [ 0.81818182, 0.18181818], [ 0.88335704, 0.11664296], [ 0.19066794, 0.80933206], [ 0.730794 , 0.269206 ], [ 0.86733889, 0.13266111]])
YAY!
Backward
cnt = 0 def backward(X, S, epsilon, T): n_obs = len(X) n_states = len(S) beta = np.zeros((n_obs + 1, n_states)) # 1 extra for initial state beta[0] = np.ones(*S.shape) beta[0] /= beta[0].sum() for k_plus_one in range(n_obs): # n-1, ..., 1 k = k_plus_one + 1 # iterating backwards yah know beta_k = beta[k] # just aliasing the pointers beta_k_plus_one = beta[k_plus_one] # beta_{k+1} x_plus_one = X[k_plus_one] # x_{k+1} for z_k in S: # for each val of z_k beta_k[z_k] = sum( # p(z_{k+1} | z_k) * p(X_{k+1} | z_{k+1}) * beta_{k+1}(z_{k+1}) T[z_k, z] * epsilon(z, x_plus_one) * beta_k_plus_one[z] for z in S # z_{k+1} ) # validating the time complexity global cnt cnt += n_states # normalize the beta over the z's at each stage # since it represents p(x_{k+1:n} | z_k) beta_k = beta_k / beta_k.sum() # finally we gotta get the probabilities of the intial state x = X[0] for z_1 in S: beta[-1][z_1] = sum(T[z_1, z] * epsilon(z, x) * beta[-2][z] for z in S) # and normalize this too beta[-1] /= beta[-1].sum() # reversing the results so that we have 0..n return beta[::-1] backward(X_wiki, S_wiki, epsilon_wiki, T_wiki)
array([[ 0.64693556, 0.35306444], [ 0.03305881, 0.02275383], [ 0.0453195 , 0.0751255 ], [ 0.22965 , 0.12185 ], [ 0.345 , 0.205 ], [ 0.5 , 0.5 ]])
Which is the wanted result! Great!
forward
and backward
algorithms
provide me with similar, but slightly different answers when we run
both of them independently on all of our observations.
BRAINFART! The forward
algorithm aims to compute
the joint distribution , while the
backward
algorithm computes the conditional , in the
case where we give the algorithms all the observations. They're not
computing the same thing, duh.
And just to validate the time-complexity:
print "Want it to be about %d; it is in fact %d" % (len(X) * len(S_wiki), cnt)
Want it to be about 400; it is in fact 20
Forward-backward
Now for bringing it all together: forward-backward.
cnt = 0 def forward_backward(pi, X, S, epsilon, T): n_obs = len(X) n_states = len(S) posterior = np.zeros((n_obs + 1, n_states)) forward_ = forward(pi, X, S, epsilon, T) backward_ = backward(X, S, epsilon, T) for k in range(n_obs + 1): posterior[k] = forward_[k] * backward_[k] posterior[k] /= posterior[k].sum() return posterior forward_backward(pi_wiki, X_wiki, S_wiki, epsilon_wiki, T_wiki)
array([[ 0.64693556, 0.35306444], [ 0.86733889, 0.13266111], [ 0.82041905, 0.17958095], [ 0.30748358, 0.69251642], [ 0.82041905, 0.17958095], [ 0.86733889, 0.13266111]])
Eureka! We got the entire posterior distribution of the hidden states given the observations!
Discussion
n
o.O
I wasn't normalizing at each step, but instead waited until I had all the values and then normalized. Which means that I would get insanely large values of alpha, and thus doom.
I'm not 100% but I think it would still be a probability distribution
in the end, if, you know, I could get to the end without overflow
error…
Example
predicted = [] posterior = forward_backward(pi, X, S, epsilon, T) for p in posterior: predicted.append(np.argmax(p))
plt.scatter(steps, X, s=25) plt.scatter(steps, zs, s=10) plt.scatter(steps, predicted[:-1], alpha=0.1, c="g") plt.title("%d samples" % n)
Here you can see the actual states plotted in blue
,
the normally distributed observations in red
, and
the predictions of the hidden states as a vague green
.
Yeah, I should probably mess with the sizes and colors
to make it more apparent..
Viterbi algorithm
Allows us to compute the posterior probability
of a sequence of states, , given some
sequence of observations,
.
Notation
i.e. the sequence of observations made, not the rv representing some
represents the sequence of observations from the
observation to the
observation
Algorithm
The main idea behind the Viterbi algorithm is to find the optimal / most probable sub-sequence at each step, and then find the optimal / most probable sub-sequence at the next step, using the previous steps optimal path.
The naive approach would be to simply obtain the probabilities
for all the different sequences of states,
given the sequence of observations
.
The issue with this approach is quite clearly the number of
possible combinations, which would be
, where
is the number of possible states.
A "dynamic programming" approach would be to attept to find a more feasible sub-problem and check if we can recursively solve these sub-problems, reducing computation by storing intermediate solutions as we go.
Wikipedia
So, let's start with the most likely intial path:
where
, i.e. probability of first state taking value
, where
Our next step is then to define
in terms of
The most likely state sequence is given by the
recurrence relations:
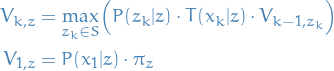
where
- probability of ???
, i.e. probability of most probable sequence of the first
elements, given that the (entire?) path ends in state
.
My interpretation
Viterbi algorithm is all about making locally optimal choices, storing this locally optimal sub-paths as you go, and reuse this computation when trying different choices of states.
You start out by the following:
Choose the initial state
s.t. you maximize the probability of observing
, i.e.
14
Now to the crucial part (and the part which makes this a dynamic programming algorithm!), we store both the probability of the sequence we've chosen so far and the states we've chosen. In this case, it's simply
and
.
Choose the next state
s.t. you maximize the probability of observing
, conditioned on already being in state
, i.e.
15
And again, we store the current path and it's probability, i.e.
and
. Important: We store this somewhere else than the first sub-path obtained, i.e.
and
.
- Perform this step until you reach the final step/observation,
i.e.
times and you end up in
.
Pretty complex, ehh? Simply choosing what seems the best at each step you take.
Now, let's think about the number of computations we got to do here.
- At each step we got to decide between
possible states, computing the probability
for each one of them. This is required so that we know which one to choose for our next state. That is; at each step we do
computations.
- We make the above choice until we reach the end, and so
we this is
steps.
This leads us to a complexity of , where
is number of possible states
is number of steps/observations
Unfortunately we're not done yet. We've performed
operations, but what if the initial probability
of the state we chose first,
, has "horrible"
probabilites as soon as you get past the first step?!
Maybe if we set
equal to some other value, we
would get a much better probability in the end?
Hence, smart as we are, we go "Okay, let's try again,
but this time we choose the second best initial state!".
And because we're running out of notation, we call this
. Yeah I know, it's pretty bad, but you get the picture.
The
denotes that this is the 2nd try until we've
either realized that it's worse than our current
,
in which case we discard the new value, or we realize it's
in fact better, and so we simply replace it, i.e.
set
, also replacing the probability.
But how do we decide that the new suggested value is better? By walking through the path again, of course! But, this time we're going to try and be smart.
- If we at some step
reach a node that we visited in our first "walk", we don't have to keep going until the end, but instead we terminate and do the following:
- Compare the probability of the sub-sequence
up until this step that we're currently looking at, with the
previous best estimate for this same sub-sequnce, i.e.
compare
to
.
If the new sub-path is more probable, then we replace our current best estimate for this sub-path, and recompute the joint conditional distribution for the rest of the path.
- Simply divide away our previous estimate, and multiply with
new and better sub-path probability:
16
- Compare the probability of the sub-sequence
up until this step that we're currently looking at, with the
previous best estimate for this same sub-sequnce, i.e.
compare
For the sake of clarification, when we use the word
sub-path, we're talking about
a sequence of states for some
, i.e.
all sub-paths start at step
, ending at some
. We're
not talking about sequences starting at any arbitrary
.
We repeat the process above for all possible initial states,
which gives us another factor of in the time-complexity.
This is due to having to perform the (possibly, see below)
number of operations we talked about earlier for each
initial state, for which there are
different ones.
This means that, at worst, we will not reach any already
known / visited sequence, and so will do all the steps
for each possible initial state. For example, consider the case
of every state only being able to transition to itself.
In such cases we will have a
time complexity of
which is still substantially
better than
, the naive approach. But as I said, this
is worst case scenario! More often we will find
sub-sequences that we already have computed, and so we won't
have to do all the
steps for every initial state!
Thus, for the Viterbi algorithm we get a time complexity of
(note Big-O, not Big-Theta)!
Implementation
First we need to set up the memoization part of the algorithm. Between each "attempt" we need to remember the following:
- At each step
, the probability of most the likely sub-path. We'll store as an array
best_sequence
, with the theelement pointing to an array of length
, holding the sequence/path of states for our best guess so far.
- At each step
, the state which was chosen by the most likely sub-path. We'll store this in an array
best_sequence
, with theelement being the probability of the sequence/path stored at
best_sequence_probas[i]
.
n_obs = len(X_wiki) n_states = len(S_wiki) best_sequence = [None] * n_obs best_sequence_probas = np.zeros(n_obs) n_obs, n_states
5 | 2 |
First we choose the "best" initial state as our
starting point, and then start stepping through.
Best initial state corresponds to maximize the
over
.
Notice how we're not actually computing real probabilities as we're not normalizing. The reason for this is simply that it is not necessary to obtain the most likely sequence.
x_1 = X_wiki[0] z_hat = S[0] z_hat_proba = epsilon_wiki(S[0], x_1) * pi_wiki[S[0]] initial_step_norm_factor = z_hat_proba for z in S_wiki[1:]: z_proba = epsilon_wiki(z, x_1) * pi_wiki[z] initial_step_norm_factor += z_proba if z_proba > z_hat_proba: z_hat = z z_hat_proba = z_proba best_sequence[0] = 1 best_sequence_probas[0] = epsilon_wiki(1, x_1) * pi_wiki[1] / initial_step_norm_factor best_sequence[0], best_sequence_probas[0]
1 | 0.18181818181818182 |
for i, x in enumerate(X_wiki): if i == 0: continue prev_sequence = best_sequence[i - 1] z_hat_prev = best_sequence[i - 1] z_hat_prev_proba = best_sequence_probas[i - 1] # initialize z_hat = S_wiki[0] z_hat_proba = T_wiki[z_hat_prev, z_hat] * epsilon_wiki(z_hat, x) step_norm_factor = z_hat_proba for z in S_wiki[1:]: z_proba = T_wiki[z_hat_prev, z] * epsilon_wiki(z, x) step_norm_factor += z_proba if z_proba > z_hat_proba: z_hat = z z_hat_proba = z_proba best_sequence[i] = z_hat best_sequence_probas[i] = z_hat_proba * z_hat_prev_proba / step_norm_factor best_sequence, best_sequence_probas
(1 0 1 0 0) | array | ((0.18181818 0.11973392 0.09269723 0.06104452 0.0557363)) |
When I started doing this, I wanted to save the sub-path at each step.
Then I realized that this was in fact not necessary, but we instead could
simply store the state at step
and the probability of the previous
best estimate for the sub-path being in state
at step
.
When we're finished, we're left with a sequence
which is the best estimate.
If you don't remember; what we did previously was storing the entire
sub-path up until the current step, i.e. at step we stored the sequence
, which is bloody unecessary. This would allow us to
afterwards obtain the best estimate for any path though, so that would be nice.
But whenever we would hit a better estimate for a previously obtained path,
we would have to step through each
element for the stored sub-paths
and replace them.
That was our first run, now we gotta give it a shot for the second best one. Since, as mentioned before, we've assumed a uniform prior, we simply pick the next state.
curr_seq = [0] curr_seq_probas = [epsilon_wiki(curr_seq[0], x_1) * pi[curr_seq[0]] / initial_step_norm_factor] for i, x in enumerate(X_wiki): if i == 0: continue z_prev = curr_seq[i - 1] z_prev_proba = curr_seq_probas[i - 1] # initialize the argmax process z_next = S_wiki[0] z_next_proba = T_wiki[z_prev, z_next] * epsilon_wiki(z_next, x) step_norm_factor = z_next_proba for z in S_wiki[1:]: z_proba = T_wiki[z_prev, z] * epsilon_wiki(z, x) step_norm_factor += z_proba if z_proba > z_next_proba: z_next = z z_next_proba = z_proba curr_seq_proba = curr_seq_probas[i - 1] * z_next_proba / step_norm_factor curr_seq.append(z_next) curr_seq_probas.append(curr_seq_proba) # check if the rest of the path has been # computed already z_hat = best_sequence[i] if z_hat == z_next: # we've computed this before, and the rest # of the path is the best estimate we have # as of right now best_seq_proba = best_sequence_probas[i] if best_seq_proba < curr_seq_proba: # the new path is better, so we gotta # replace the best estimate best_sequence[:i + 1] = curr_seq # and the preceding probabilities best_sequence_probas[:i + 1] = curr_seq_probas # then we have to recompute the probabilities # for the rest of the best estimate path for j in range(i + 1, n_obs): best_sequence_probas[j] = curr_seq_proba * \ best_sequence_probas[j] \ / best_seq_proba print "We found a better one! Current is %f, new is %f" \ % (best_seq_proba, curr_seq_proba) # since we've already computed the sub-path after this step # given the state we are currently in, we terminate break curr_seq, curr_seq_probas
0 | 0 |
0.8181818181818181 | 0.7470355731225296 |
best_sequence, best_sequence_probas
(0 0 1 0 0) | array | ((0.81818182 0.74703557 0.57835012 0.38086471 0.34774604)) |
This looks very good to me!
def viterbi(pi, X, S, epsilon, T): n_obs = len(X) # initialize best_sequence = [None] * n_obs best_sequence_probas = np.zeros(n_obs) x_1 = X[0] initial_marginal = sum(epsilon(z, x_1) * pi[z] for z in S) sorted_S = sorted(S, key=lambda z: epsilon(z, x_1) * pi[z], reverse=True) for z_1 in sorted_S: curr_seq = [z_1] curr_seq_probas = [epsilon(z_1, x_1) * pi[z_1] / initial_marginal] for i, x in enumerate(X): if i == 0: continue z_prev = curr_seq[i - 1] z_prev_proba = curr_seq_probas[i - 1] # Max and argmax simultaenously # initialize the argmax process z_next = S[0] z_next_proba = T[z_prev, z_next] * epsilon(z_next, x) step_marginal = z_next_proba for z in S[1:]: z_proba = T[z_prev, z] * epsilon(z, x) step_marginal += z_proba if z_proba > z_next_proba: z_next = z z_next_proba = z_proba # Memoize our results curr_seq_proba = z_prev_proba * z_next_proba / step_marginal curr_seq.append(z_next) curr_seq_probas.append(curr_seq_proba) # check if the rest of the path has been computed already z_hat = best_sequence[i] if z_hat == z_next: # We've computed this before, and the rest # of the path is the best estimate we have. # Thus we check if this new path is better! best_seq_proba = best_sequence_probas[i] if best_seq_proba < curr_seq_proba: # replace the best estimate sequence best_sequence[:i + 1] = curr_seq # and the corresponding probabilities best_sequence_probas[:i + 1] = curr_seq_probas # recompute the probabilities # for the rest of the best estimate path for j in range(i + 1, n_obs): best_sequence_probas[j] = curr_seq_proba * \ best_sequence_probas[j] \ / best_seq_proba print "We found a better one at step %d!" \ "Current is %f, new is %f" \ % (i, best_seq_proba, curr_seq_proba) # since we've already computed the sub-path after this step # given the state we are currently in, we terminate break # gone through all the observations for this state if i == n_obs - 1: best_seq_proba = best_sequence_probas[i] if best_seq_proba < curr_seq_proba: best_sequence = curr_seq best_sequence_probas = curr_seq_probas return best_sequence, best_sequence_probas
viterbi(pi_wiki, X_wiki, S_wiki, epsilon_wiki, T_wiki)
0 | 0 | 1 | 0 | 0 |
0.8181818181818181 | 0.7470355731225296 | 0.5783501211271196 | 0.3808647139129812 | 0.3477460431379394 |
Discussion
Using numpy.ndarray
for curr_seq
I'm actually not sure whether or not this would speed up things.
The way Python list
work provides us with a ammortized constant
time append
method. This means that we can potentially save loads of
memory compared to having to preallocate memory for n_obs
elements
using numpy.ndarray
. Most of the time we won't be walking through
the entire sequence of observations, and so most of the memory allocated
won't even be used.
Nonetheless I haven't actually made any benchmarks, so it's all speculation. I just figured that it wasn't worth trying for the reason given here.
Python class implementation
Should this even be a class
? I think it sort of makes sense, considering
it's kind of a model of a state machine. And so modelling it in a imperative
manner makes sense in my opinion.
Would it be a good idea to implement all of these as a
staticmethod
, and then have the instance specific method
simply call the staticmethod
? This would allow us to choose
whether or not we actually want to create a full instance of
a HiddenMarkovModel
or not.
Naaah, we simply have functions which take those arguments
and then have the class
HiddenMarkovModel
call those methods.
Methods:
forward
backward
forward-backward
viterbi
Properties:
posterior
epsilon
andemission_probabilities
T
andtransition_probabilities
pi
andinitial_probabilities
S
andstates
X
andobservations
class HiddenMarkovModel: """ A Hidden Markov Model (HMM) is fully defined by the state space, transition probabilities, emission probabilities, and initial probabilities of the states. This makes it easier to define a model, and then rerun it on different observations `X`. Parameters ---------- pi: array-like floats, 1d Initial probability vector. S: array-like ints, 1d Defines the possible states the system can be in. epsilon: callable(z, x) -> float Takes current state :int:`z` and observation :float:`x` as argument, returning a :float: representing the probability of seeing observation :float:`x` given the system is in state :int:`z`. T: array-like floats, 2d Matrix / 2-dimensional array where the index `[i][j]` gives us the probability of transitioning from state `i` to state `j`. X: array-like (optional) Represents the observations. This can be a array-like structure of basically anything, as long as :callable:`epsilon` can handle the elements of `X`. So observations can be scalar values, vectors, or whatever. """ def __init__(self, pi=None, S=None, epsilon=None, T=None, X=None): self.pi = pi self.S = S self.epsilon = epsilon self.T = T self.X = X # some aliases for easier use for others self.initial_probabilities = self.pi self.states = self.S self.emission_probabilities = self.epsilon self.transition_probabilities = self.T self.observations = self.X self.posterior = None self.optimal_path = None def forward(self, X=None): if X is None: X = self.X return forward(self.pi, X, self.S, self.epsilon, self.T) def backward(self, X=None): if X is None: X = self.X return backward(X, self.S, self.epsilon, self.T) def forward_backward(self, X=None): if X is None: X = self.X n_obs = len(X) n_states = len(S) posterior = np.zeros((n_obs + 1, n_states)) forward_ = self.forward(X) backward_ = self.backward(X) for k in range(n_obs + 1): posterior[k] = forward_[k] * backward_[k] posterior[k] /= posterior[k].sum() # Should we actually set it, or leave that to the user? self.posterior = posterior return posterior def viterbi(self, X=None): if X is None: X = self.X self.optimal_path = viterbi(self.pi, X, self.S, self.epsilon, self.T) return self.optimal_path
hmm = HiddenMarkovModel(pi_wiki, S_wiki, epsilon_wiki, T_wiki) hmm.viterbi(X_wiki) hmm.forward_backward(X_wiki) hmm.optimal_path
0 | 0 | 1 | 0 | 0 |
0.8181818181818181 | 0.7470355731225296 | 0.5783501211271196 | 0.3808647139129812 | 0.3477460431379394 |